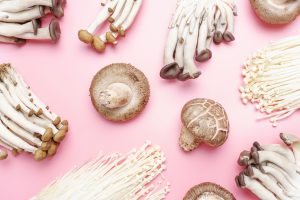
Do you prefer shiitakes? Cremini? Portabellos? Or are plain old white button mushrooms your favorite? In a quirky twist in AI research, a multi-institutional team of computer scientists asked people to choose the tastiest mushrooms and explain their picks. The researchers weren’t out simply to find the best pizza topping or ingredient for a killer risotto: Their goal is to create artificial intelligence systems that not only grasp the nuances of human decision-making but also make choices that align with human values.
“AI systems must understand what their human users find important to provide personalized experiences, collaborate better with people, and make ethical decisions,” says Tianmin Shu, an assistant professor in the Whiting School of Engineering’s Department of Computer Science and a research team member. “Our machine learning framework goes beyond simply asking users which choice they prefer, delving into why they made that particular choice. This provides richer information from which an AI model can learn.”
The team’s work will appear at the 41st International Conference on Machine Learning, to be held in Vienna, Austria from July 21 to 27.
The framework, called “pragmatic feature preferences,” asks users to explain why they choose one option over another, giving insight into their preferred features in a particular choice. The researchers validated the applicability of their method with an online behavioral experiment in which study participants were tasked with selecting the tastiest of several mushrooms based on predetermined criteria such as size, shape, and color.
When making their selections, participants were asked to evaluate mushrooms using one of three types of preference queries, each representing a distinct AI learning approach. The baseline method, called reinforcement learning from human feedback, is commonly used in training large language models. In this method, users simply chose which mushroom they preferred based on the tastiness criteria provided as part of the study. The second approach—which the researchers termed “feature preference querying”—directed participants to select which of two mushrooms they preferred for each of six mushroom characteristics. The third method, representing the researchers’ new framework, asked participants to provide detailed written descriptions of what a delicious mushroom looks like when they made their choices, revealing the most important features they associated with mushroom tastiness.
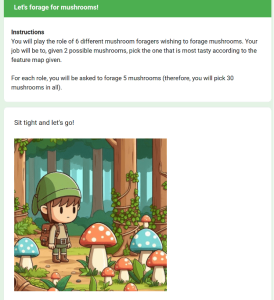
Mushroom foraging task user interface. Image Credit: Bing Image Creator
The team compared AI models trained on each type of user answer and found that the model trained on its method outperformed the others, more accurately predicting and representing human preferences—and doing so with fewer human examples.
“By leveraging feature-level preferences from human feedback, our approach can significantly outperform standard reward learning methods,” says Shu.
The researchers also verified that asking users to provide more detailed explanations didn’t significantly increase their perceived effort or frustration with the mushroom ranking task.
“Our method allows us to grab more information per user interaction without having users spend significantly more effort giving feedback,” says Boston University’s Yuying Sun, another research team member. “Systems with this framework will be able to quickly adapt to individual users’ preferences to provide better, more personalized assistance.”
The team plans to extend its framework to learn even more from feedback that goes beyond written descriptions.
“People may talk about their preferences for certain items—in spoken language—while pointing to parts of the items that are important—with physical gestures,” says Shu. “Such multimodal feedback can enable more sophisticated human reward learning and allow AI systems to engage in more natural interactions with human users in the real world.”
Additional contributors to this work include lead author Andi Peng, a PhD student at the Massachusetts Institute of Technology’s Computer Science and Artificial Intelligence Laboratory, and David Abel, a senior research scientist at Google DeepMind.