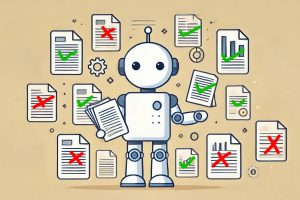
Researchers in the Department of Computer Science and the Center for Language and Speech Processing are tackling a crucial challenge in our hyper-expanding digital age: safeguarding question-answering systems that extract information from websites. These systems are vulnerable to manipulation by malicious actors who inject false information, threatening their reliability.
The researchers’ new Confidence from Answer Redundancy method works by searching for and comparing multiple sources that address the same question, determining which response is most likely to be correct even when some of the information has been falsified or otherwise altered.
“Our approach significantly reduced the dissemination of false information in user outputs, doubling accuracy in certain scenarios, and proved effective across all levels of malicious content, from 1% to 100% of poisoned articles,” says PhD student Orion Weller, one of the researchers on the team. “Our method distinguishes high-confidence responses from uncertain ones, prompting the system to consider alternative queries when confidence is low, thus bolstering resilience against adversarial attacks.”
The team’s results were published and presented at the 18th Conference of the European Chapter of the Association for Computational Linguistics this spring.
Weller and his team used advanced computational models to generate a broad spectrum of questions that were closely related, seeking answers from diverse sources. This reduced reliance on any single source that included potentially compromised data.
To test how well their method worked, the researchers used benchmarks like Natural Questions—a large Google dataset comprising real queries that people have asked the search engine—and TriviaQA—another large-scale dataset designed to train AI models to answer trivia questions—on simulated scenarios where Wikipedia pages answers had been maliciously altered. Their approach proved effective, identifying correct answers 20% more often.
Weller warns of the growing threats posed by combined language models and retrieval systems—which access and incorporate information from external sources—in that they might accidentally spread disinformation injected by malicious website owners.
“This was evident when Google’s new AI-generated answers replaced traditional ranked search results, but these responses were often wrong. Experts warned that this feature could spread misinformation and bias, endangering users in critical situations. Google has made some fixes to handle these issues, but concerns remain about the impact on information accuracy and the disruption of traffic to traditional websites,” Weller says.
Despite promising results, researchers acknowledged limitations in topics less represented online or less scrutinized, emphasizing the need for ongoing collaboration to refine defenses against evolving online threats. The team plans to share its tools and findings widely, promoting joint efforts to enhance the reliability and security of question-answering systems.
Other contributors to the study include PhD students Aleem Khan and Nathaniel Weir; Benjamin Van Durme, an associate professor of computer science; and Dawn Lawrie, a senior research scientist at the Human Language Technology Center of Excellence.