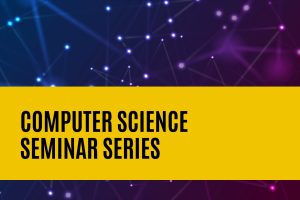
Lunch will be served at 12 p.m. The seminar will begin at 12:15 p.m.
Abstract
In this talk, Eli N. Weinstein studies how to efficiently manufacture samples from a generative model in the real world. He shows how computational methods for approximate sampling can be adapted into experimental design methods for efficiently making samples in the laboratory. He also develops tools to rigorously evaluate the quality of manufactured samples, proposing nonparametric two-sample tests with strong theoretical guarantees and scalable algorithms. He applies these methods to DNA synthesis, since the cost of DNA synthesis is considered a fundamental technological driver of progress of biology and biomedicine. He demonstrates manufacturing ~10^17 samples from a generative model of human antibodies at a sample quality comparable to that of state-of-the-art protein language models. These samples cost roughly a thousand dollars to make (~$10^3), while using previous methods they would cost roughly a quadrillion dollars (~$10^15).
Speaker Biography
Eli N. Weinstein is a postdoctoral research scientist at Columbia University advised by David Blei. He also serves as the director of machine learning research at Jura Bio, a biotechnology startup focused on genetic medicine. Weinstein’s research is in probabilistic machine learning, with an emphasis on causal inference and experimental design. His applied work focuses on biology, especially therapeutics. He completed his PhD in biophysics at Harvard University in 2022, advised by Debora Marks and Jeffrey Miller and supported by a Hertz Foundation Fellowship. Previously, he received an AB in chemistry and physics with highest honors, also from Harvard, advised by Adam Cohen. His work has been published at venues including the Conference and Workshop on Neural Information Processing Systems, the International Conference on Machine Learning, the International Conference on Artificial Intelligence and Statistics, and the Journal of Machine Learning Research, and has received Best Paper Awards from the New England Statistical Society in 2021 and 2023 and the Molecular Machine Learning Conference in 2024.