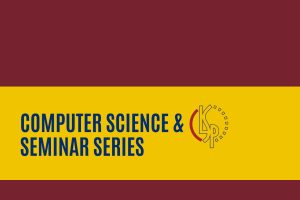
Abstract
Recent advances in large language models have achieved remarkable results across a wide range of natural language processing applications, including text classification, summarization, machine translation, and dialogue systems. As LLMs grow increasingly capable, the need to control their generation process becomes more pressing, particularly for high-stakes applications that demand reliable outputs adhering to specific guidelines or creative outputs within defined boundaries. However, the dominant auto-regressive paradigm—training models to predict the next word based on prior context—poses significant challenges for enforcing structural or content-specific constraints.
In this talk, Nanyun “Violet” Peng will present her recent work on controllable natural language generation that moves beyond the conventional auto-regressive framework to enhance both the reliability and creativity of generative models. She will introduce controllable decoding-time algorithms that guide auto-regressive models to better align with user-specified constraints. Additionally, she will discuss a novel insertion-based generation paradigm that breaks away from the limitations of auto-regressive methods. These approaches enable more reliable and creative outputs, with applications spanning creative writing, lexical-controlled generation, and commonsense-compliant text generation.
Speaker Biography
Nanyun “Violet” Peng is an associate professor of computer science at the University of California, Los Angeles. Her research focuses on controllable and creative language generation, multilingual and multimodal models, and the development of automatic evaluation metrics, with a strong commitment to advancing robust and trustworthy AI. Her work has been recognized with honors such as an Outstanding Paper Award at the Annual Conference of the North American Chapter of the Association for Computational Linguistics in 2022, three Outstanding Paper Awards at Empirical Methods in Natural Language Processing in 2024, oral paper selections at the 2022 Conference and Workshop on Neural Information Processing Systems and the 2023 International Conference on Machine Learning, and several Best Paper Awards at workshops affiliated with premier AI and NLP conferences; she was also featured in the 2022 International Joint Conferences on Artificial Intelligence Early Career Spotlight. Peng’s research has received support from prestigious funding sources, including an NSF CAREER Award, a National Institutes of Health R01 grant, grants from DARPA and the Intelligence Advanced Research Projects Activity, and multiple industrial research awards. She received her PhD from the Center for Language and Speech Processing at the Johns Hopkins University.