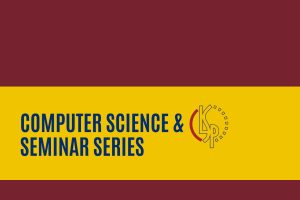
Abstract
Over the past decades, machine learning has primarily relied on labeled data, with success often depending on the availability of vast, high-quality annotations and the assumption that test conditions mirror training conditions. In contrast, humans learn efficiently from conceptual explanations, instructions, rules, and contextual understanding. With advancements in large language models, AI systems can now understand descriptions and follow instructions, paving the way for a paradigm shift.
This talk explores how teaching machines through language and rules can enable AI systems to gain human trust and enhance their inclusivity, robustness, and ability to learn new concepts. Kai-Wei Cheng will highlight his journey in developing vision-language models capable of detecting unseen objects through rich natural language descriptions. Additionally, he will discuss techniques for guiding the behavior of language models and text-to-image models using language. He will also describe his efforts to incorporate constraints to control language models effectively. Finally, he will conclude his talk by discussing future directions and challenges in building trustworthy language agents.
Speaker Biography
Kai-Wei Chang is an associate professor in the Department of Computer Science at the University of California, Los Angeles and an Amazon Scholar at Amazon AGI. His research focuses on trustworthy AI and multimodal language models. He has published extensively in natural language processing and machine learning, with his work widely recognized through multiple paper awards at top conferences, including Empirical Methods in Natural Language Processing (EMNLP), the Annual Meeting of the Association for Computational Linguistics (ACL), the ACM SIGKDD Conference on Knowledge Discovery and Data Mining, and the Conference on Computer Vision and Pattern Recognition. In 2021, Chang was honored as a Sloan Fellow for his contributions to trustworthy AI as a junior faculty member.
Recently, Chang was elected as an officer of the ACL Special Interest Group on Linguistic Data & Corpus-based Approaches to Natural Language Processing, the organizing body behind EMNLP, and will serve as its vice president in 2025 and president in 2026. He is an associate editor for leading journals such as the Journal of Artificial Intelligence Research, the Journal of Machine Learning Research, Transactions of the Association for Computational Linguistics, and the ACL Rolling Review. He also served as an associate program chair at the Thirty-Seventh AAAI Conference on Artificial Intelligence and as senior area chair for most NLP, machine learning, and AI conferences. Since 2021, Kai-Wei has organized five editions of the Trustworthy NLP Workshop at ACL, a platform that fosters research on fairness, robustness, and inclusivity in NLP. Additionally, he has delivered multiple tutorials on topics such as Fairness, Robustness, and Multimodal NLP at EMNLP (2019, 2021) and ACL (2023). Chang received his PhD from the University of Illinois at Urbana-Champaign in 2015 and subsequently worked as a postdoctoral researcher at Microsoft Research in 2016. For more details, visit kwchang.net.