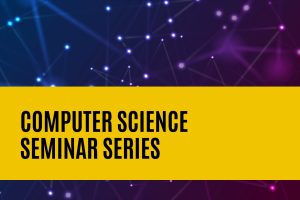
Refreshments are available starting at 10:30 a.m. The seminar will begin at 10:45 a.m.
Abstract
Bilevel optimization has emerged as a foundational framework in modern machine learning for developing principled computational tools across diverse domains, including meta-learning, automated ML, reinforcement learning, and robotics. This talk will explore recent advancements in bilevel optimization algorithms and their applications in machine learning. In the first part, Kaiyi Ji will introduce several efficient implicit gradient-based algorithms featuring flexible double- and single-loop structures. He will then present a novel adaptive tuning-free approach that significantly reduces hyperparameter tuning efforts while maintaining strong convergence guarantees. For the more challenging scenario involving non-unique lower-level solutions, Ji will discuss penalty-based methods that ensure provable convergence using only first-order gradient information. The second part of the talk will focus on two practical applications of bilevel optimization: coreset selection for machine learning and imperative learning for optimization and robotics. Finally, Ji will discuss promising directions for future research in theoretical and applied bilevel optimization and in other areas such as multi-objective learning and continual learning.
Speaker Biography
Kaiyi Ji is an assistant professor in the Department of Computer Science and Engineering (CSE) of the University at Buffalo and an affiliated faculty member with university’s Institute for Artificial Intelligence and Data Science. He was a postdoctoral research fellow in the Department of Electrical Engineering and Computer Science at the University of Michigan, Ann Arbor in 2022. Ji earned his PhD in electrical and computer engineering from the Ohio State University in 2021 and was a visiting student research collaborator in the Department of Electrical Engineering at Princeton University. He completed his BS degree at the University of Science and Technology of China in 2016. Ji’s research lies at the intersection of optimization, machine learning, and communication networks, spanning both theoretical foundations and practical applications. His current interests include bilevel optimization, multi-objective and multi-task learning, and continual learning, with applications in deep learning, communication, and recommendation systems. He has received several honors, including a CSE Junior Faculty Research Award, a UB Presidential Fellowship, and multiple NSF awards.