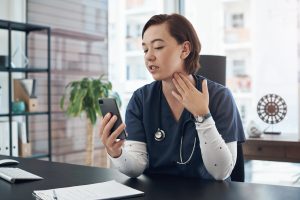
A new study by a multidisciplinary team of Johns Hopkins researchers found that while AI-powered systems can improve clinicians’ diagnostic accuracy during telehealth visits, doctors still don’t fully trust algorithms to screen patients—highlighting the enduring need to improve human-AI collaboration. The team’s findings appeared last week in Nature Communications Medicine.
“Physicians do better with AI assistance, yet they still hesitate to alter their practices significantly as a result. For example, they will still frequently ask telehealth patients to visit the clinic for definitive testing,” said team member Mathias Unberath, John C. Malone Associate Professor of Computer Science at the Whiting School of Engineering. “This shows there is room for improvement in fostering trust to enhance human-machine collaboration.”
In their study, the researchers collaborated with CurieDx, a smartphone-based AI system developed by Unberath and colleague Therese Canares of the School of Medicine to examine explainable AI’s potential to enhance clinicians’ trust in AI-driven diagnostic tools. Specifically, they delved into how doctors use and perceive the system’s explanations of its strep throat diagnoses, which rely on analyzing smartphone images of users’ throats.
To do this, the researchers created mock-ups featuring various techniques the AI system might use to explain its diagnoses, including a method that highlighted key visual features of throat images and one that provided examples of images that the system had already analyzed and accurately diagnosed as either strep or not.
They then had primary care and emergency medicine providers review the mock-ups, measuring the providers’ agreement with CurieDx’s resulting diagnostic recommendations, the clinicians’ perceived trust in the system, and how the explanations for those decisions influenced the providers’ ultimate diagnostic recommendations.
“We found that explaining by example was the most promising method of explainable AI in this instance,” said team member and lead author Catalina Gomez, a PhD student in the Department of Computer Science.
The researchers theorized that this type of explanation was the most successful because it most closely mirrors human clinical reasoning, which involves incorporating prior experience in the analysis of a patient’s condition.
“This kind of explanation improved the accuracy of the clinicians’ decisions. The providers also trusted the AI-generated predictions just as much as they trusted the diagnoses produced by their customary clinical prediction rule, which acted as our baseline,” says Gomez.
Even so, the clinicians in the study often felt it was necessary to ask patients diagnosed remotely to visit the clinic for a follow-up.
“This opens the dialogue to examine clinical workflows that incorporate AI screening tools,” says Canares, noting the complexity of factors that contributes to medical decision-making, including patients’ risk factors, clinical protocols, and professional guidelines.
The team plans to continue exploring explainable AI methods to help users better understand how AI-powered systems work and further enhance user trust in those systems.
In the meantime, this research is helping CurieDx promote explainability, increase trust, and hopefully provide a little ease of mind to patients at home with a sore throat.
Additional co-authors of this work include Brittany-Lee Smith, A&S ’22 (MS), and Alisa Zayas, Med ’23 (MS). This work was supported by the Bisciotti Foundation Translational Fund at the Johns Hopkins University.