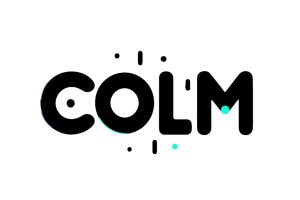
A team from Johns Hopkins won an Outstanding Paper Award at the 2024 Conference on Language Modeling, held October 7-9 in Philadelphia, for their investigation into whether the data used to train large language models is up to date. The authors of the winning paper, “Dated Data: Tracing Knowledge Cutoffs in Large Language Models,” are Jeffrey Cheng, a master’s student of computer science; PhD students Marc Marone and Orion Weller; Dawn Lawrie, a senior research scientist in the Human Language Technology Center of Excellence; and Daniel Khashabi and Benjamin Van Durme, both faculty in the Whiting School of Engineering’s Department of Computer Science and members of the Center for Language and Speech Processing.
The team’s paper explores the “cutoff dates” used by popular LLMs like ChatGPT, which claim to inform users about the freshness of their information. However, the researchers questioned whether these dates accurately reflect the most recent data used by the models.
“For example, a model could have news articles from 2024 in its training data, but only have scientific papers from up to 2022,” explains Marone. “A single cutoff date doesn’t capture those subtleties.”
The researchers sought to identify discrepancies between the cutoff date given by LLMs’ creators and the actual information used by the models. They designed a probe to track which version of an often-changing resource—such as a Wikipedia page that gets updated or a curated collection of news articles—an LLM is the most familiar with, signifying that model’s effective cutoff date.
“For example, a model might be labeled with a cutoff date of October 2023, but our method could show that its effective cutoff is more closely aligned to March 2020,” says Marone.
Using this technique, the researchers determined that many models had differing actual and claimed cutoff dates—often due to LLMs having gathered information from much older versions of websites than currently exist today. The researchers contend that this is problematic for users because LLMs might have up-to-date information about one topic but out-of-date information about another.
“Model builders need to carefully consider and account for issues like this when designing and assembling datasets,” says Marone. “Which is exactly the reason we identify such issues in the first place—it’s all part of our ongoing effort to make LLM systems more reliable and trustworthy.”