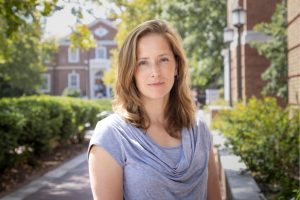
Jessica Sorrell joins the Johns Hopkins University as an assistant professor of computer science.
Sorrell completed her PhD at the University of California, San Diego. Prior to joining Johns Hopkins, she was a postdoctoral researcher at the University of Pennsylvania.
Tell us a little bit about your research.
I work on the theoretical foundations of machine learning, particularly questions related to algorithmic properties of privacy, fairness, and replicability. These properties aim to mitigate potential harms arising from the use of models in high-stakes contexts. I design algorithms satisfying formal notions of these properties—and study the relationships between them—to better understand the potential and limitations for responsible ML.
Tell us about a project you are excited about.
While pursuing my PhD, in joint work with my advisor Russell Impagliazzo, Rex Lei, and Toni Pitassi, we introduced a formal notion of replicability for learning algorithms. At a high level, replicable learning algorithms ensure that outputs of an experiment can be replicated by another team of researchers following the same experimental design, but using their own data. We’ve shown that there are interesting connections between replicable algorithms and privacy-preserving algorithms, but we don’t fully understand the limits of these connections yet. For instance, it’s sometimes the case that an efficient privacy-preserving algorithm can be transformed into an efficient replicable algorithm, but we’ve also proven results showing that, under reasonable assumptions, this can’t always be the case. I’m currently working on a project that would further our understanding of when exactly privacy-preserving algorithms can be transformed into replicable ones.
Why this? What drives your passion for your field?
I’m interested in this project in particular, because no matter how it turns out, there will be interesting consequences. If we’re able to broaden the class of tasks for which we know privacy-preserving algorithms can be efficiently transformed into replicable ones, we won’t only get new replicable algorithms—we’ll also get new privacy-preserving ones, as well! On the other hand, if we show that the class of tasks can’t be broadened in the way we hope, this in turn has implications about the limits of efficient algorithms for an interesting sampling problem. It’s a nice situation to be in as a theorist, when there are interesting consequences for proving or refuting your conjecture.
What classes are you teaching?
This spring I’m teaching Theory of Replicable Machine Learning. This course will introduce the notions of replicability, differential privacy, and other stability notions that have proven useful in guaranteeing statical validity of adaptive data analyses. We’ll discuss the contexts in which these similar but distinct stability notions arose, study the connections and separations between them, and see how they can be applied in the ML pipeline for tasks such as hyperparameter tuning.
Why are you excited to be joining the Johns Hopkins Department of Computer Science?
I draw a lot of motivation for my work from real-world problems arising in ML. As new faculty at Johns Hopkins, I plan to not only collaborate with the awesome theory faculty in the department, but also some of the many researchers working at the intersection of ML and other disciplines, like medicine, language, and policy. My hope is that working with and learning from researchers who are more “in the trenches” of ML applications than myself will inform and shape the kinds of theoretical models I study and the perspectives that I take on problems. I’m also really excited about the new Data Science & AI Institute—I think it will facilitate a lot of discussion at Hopkins around charting a responsible way forward for AI engineering, adoption, and regulation, and I’m very happy to be here for it.
Besides your work, what are some of your other hobbies and passions?
Outside of work, I like to practice circus arts, specifically aerial silks and aerial hoop. Since moving to Baltimore, I’ve been taking classes on both apparatuses at In the Dark Circus Arts, which is a great gym!