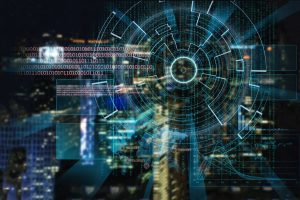
Malone researchers presented a paper titled “Deep Labeling of fMRI Brain Networks Using Cloud Based Processing” at the 17th International Symposium on Visual Computing (ISVC’22), which took place in San Diego, CA, October 3-5, 2022. The research was a collaboration between the Malone Center for Engineering in Healthcare, the Johns Hopkins Department of Radiology and Radiological Science, and Microsoft Research.
Meet the Research Team/Authors:
- Craig K. Jones, Assistant Research Professor, Computer Science
- Haris Sair, Associate Professor, Radiology and Radiological Sciences
- Sejal Ghate, Biomedical Engineering PhD student
- Alberto Santamaria-Pang, Principal Applied Data Scientist, Microsoft Research
- Ivan Tarapov, Senior Program Manager, Microsoft Research
Research Overview
Resting state fMRI (rs-fMRI) is an imaging modality which reveals brain activity localization through signal changes, in what is known as Resting State Networks (RSNs). In a clinical setting, identifying RSNs can help surgeons better plan for brain surgery; however, labeling these networks is time consuming and requires considerable medical expertise. Recognizing this, the team developed a deep learning model which incorporates image processing of rs-fMRI in a cloud-based workflow to automate the classification of RSNs with a >98% accuracy.
Significance of Research
The team compared the performance of three neural network architectures to perform classification of deeper RSNs representing over 50 functional brain regions: a multilayer perceptron (MLP) network, a 2D projection-based CNN, and a fully 3D CNN classification networks. The multilayer perceptron (MLP) network provided the fastest inference times with a testing accuracy of 100%.Furthermore, the team efficiently processed rs-fMRI image data by integrating standardized medical imaging libraries for a large cohort in a scalable cloud-based environment. This step is critical when needed to apply and optimize machine learning algorithms at large scale in realistic scenarios. This study provides a foundation for an end-to-end rs-fMRI processing and classification pipeline which can be extended to more robust multi-label/hierarchical classifications in the future.
Research Highlights
- Demonstrated a novel approach to producing an objective and automated algorithm for classifying RSNs in fMRI data
- The results indicate that all three neural networks in the study could be used as an accurate classifier to classify the RSNs, showcasing greater than 98% testing accuracy in all models.
- To the best of the author’s knowledge, this is the first study that performed classification on over 50 RSNs and that will enable the inclusion of a hierarchy in the labeled networks.
- The team plans to make their pipeline architecture code and methods public for further reproducibility.
Research Image:
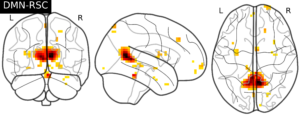
Orthogonal glass brain views in three planes demonstrate an area of high signal in the deep parietal lobe corresponding to the retrosplenial cortex, a key component of the default mode network.